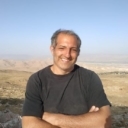
In this SafetyDetectives interview with Angèle Barbedette of Sightengine, we delve into the expertise of Sightengine, an AI company specializing in automatic content moderation. They offer a comprehensive solution for moderating various content types, including images, videos, and text, with a focus on customization and speed. Let’s explore the key insights she shared about Sightengine’s capabilities and the challenges in today’s digital content landscape.
Hi Angèle, thank you for your time today. Can you tell me a little about your background, and your current role at Sightengine?
Hi Shauli, thank you for inviting Sightengine to this interview. I’ve been working at Sightengine for a year and a half as an analyst. My work focuses on various topics such as improving our automated moderation products, contributing to the underlying technology, gathering feedback and writing documentation and material. I have a linguistics and computational linguistics background so I am used to working with text and speech. What I do at Sightengine is much more broader since I deal with all types of content, including visual content.
Can you explain what are Sightengine’s specialties?
Sightengine is an AI company that specializes in automatic content moderation in any type of content such as images, live or stored videos or text. Our focus has historically been on visual moderation. Sightengine provides over 60 moderation categories ranging from standard ones to more advanced ones. They can be combined with dozens of different context labels depending on what the user expects to be safe or problematic on its platform.
Automatic content moderation is a task that is much more complex than traditional scene classification or object detection as our models need to understand the meaning and the intent behind an image or a video and the emotions it conveys in order to correctly detect any bad or unwanted content. To do so, models learn to recognize the theme and focus of the content but also the context. This way, using what the models can flag, we are able to propose several combinations of categories and contexts so that our users can create their own moderation rules. As an example, users can decide to reject images and videos with a female taking a selfie in bikini in her bedroom, or a male showing off his abs in his bathroom, while at the same time allowing bikinis and bare chests in beach or pool contexts, and allowing males to have slightly open shirts. Other users might decide that bikinis and torsos are always ok or always problematic, or ok up to a certain type of framing or zoom. This really is the user’s choice.
Nudity detection is not the only area where customization might be needed: for weapon detection for instance, rules might differ depending on the type of weapon (it could be a firearm, a knife, etc.), the person carrying the weapon (it could be a child, an adult, someone from the police, etc.) or the location and context (public places are certainly more problematic than private homes or places where it is common to see firearms such as shooting ranges, wilderness, gun stores). Sightengine really tries to provide a full range of concepts to provide its users with a wide scope of options.
How quickly can the system detect and act upon inappropriate content?
Our solution was built to be fast. To give you an idea, images sent to the API to be checked are usually processed within a few hundreds of milliseconds. It means that users get an immediate response indicating what was detected in the content they sent.
Then, it is up to them to decide what to do with the content. They can decide to keep it on their platform, to delete it, to sanction the user who submitted the content (by using temporary or permanent ban for instance) or to educate and encourage positive behavior. Sightengine takes no part in the decision and has no direct influence on the content. We simply detect potentially harmful content and inform the customer.
What are the key challenges and complexities involved in content moderation in today’s digital landscape?
As mentioned earlier, automatic content moderation is a very challenging task.
First, real-life situations are a lot more complicated than they appear. Bad content is sometimes difficult to detect, even for a human moderator. Implicit intentions are very common in texts for instance: did the user really say that? Was it a joke? Or does this insulting word actually have another, safe meaning? And it is the same for images and videos: how do we make the difference between pills, syringes and actual recreational drugs? How do we know for sure that this sign is a hateful sign? Is this a real firearm or a toy? Is the person holding this knife cooking or threatening someone? As you can imagine, we at Sightengine have been working on these questions and we provide clear documentation detailing what we detect and how customers can create their own custom rules to choose what is acceptable or not.
Another challenge is the constant changes and new symbols or words that appear in user-generated content. As language is constantly evolving, new words, expressions, slangs, acronyms and abbreviations that are sometimes problematic are created, especially among teenagers and young adults. There are also existing words that get new meanings, which make them even more challenging to take into account. Regarding images and videos, new symbols appear very often. Some are safe, others are hate symbols that are very offensive, and it is sometimes hard to tell the difference without resources defining these new symbols. Content moderation requires us to always stay aware of potential new categories of content to teach our models.
We also need to be very cautious about the new ways users find to try to bypass moderation systems. We find a lot of these in texts. It is very frequent to deal with weird characters replacing usual characters, characters that have been inserted within words, leet speak or spellings that have intentionally been changed to evade basic filters. Similar challenges exist with images and videos, users are very creative when it comes to hiding the intent of visual content. These kinds of obfuscations are fully managed by our models and constantly updated.
How does Sightengine handle different types of content, such as text, images, and videos?
Our API can handle any user-generated content. We have specific detection models for image and video moderation and other models for text moderation.
Visual models cover any type of unwanted content we can find in images and videos, such as 8 different levels of nudity, weapons, substances like alcohol, tobacco, medical and recreational drugs, money, gambling, violence, graphic content and hate symbols. We can detect minors, which is a very important feature to keep platforms safe. All these categories can be associated with contextual information to further refine and customize the moderation. Also, some of our models are specialized in detailing information about the quality of the image or the video. We try to be as exhaustive as possible.
When it comes to text moderation, we offer two options that can be combined: classification models based on deep learning that are great to interpret full sentences and understand linguistic subtleties, and therefore moderate text based on semantic and in-context meaning, and ruled-based pattern matching algorithms that are great to flag specific words or phrases, even when these are heavily obfuscated. With these two, you get a total of approximately 20 categories, including personal details and URLs detection.
How does content moderation vary when applied to different types of platforms (e.g., social media, e-commerce, gaming, news websites)
By working with a wide range of customers over the past 10 years, we have been able to build a pretty exhaustive set of models that covers almost any trust and safety need platforms may have. Of course, content moderation always varies when applied to different types of platforms, and we like to work hand-in-hand with our customers to make sure we do not miss anything.
Gaming activities are a good example of needs and expectations that can be very specific, especially when it comes to video games featuring weapons. Platforms might authorize clips from video games where a firearm is visible but prohibit images and videos where there is a real gun. Because of that, we developed a proprietary model capable of differentiating illustrations from photography. Illustrations include video games images but also any image that does not look like a natural photo such as a drawing, a painting or a logo for instance.
This is just an example, there are many other situations that would require having even finer categories, and Sightengine provides most of them.